Machine Learning
Machine Learning on Google Cloud
Transform the way you approach business challenges with machine learning! This five-day course will show you how ML can tackle complex problems and introduce you to Vertex AI, BQML, and Dataflow, the essential tools that power modern AI applications
5 Days
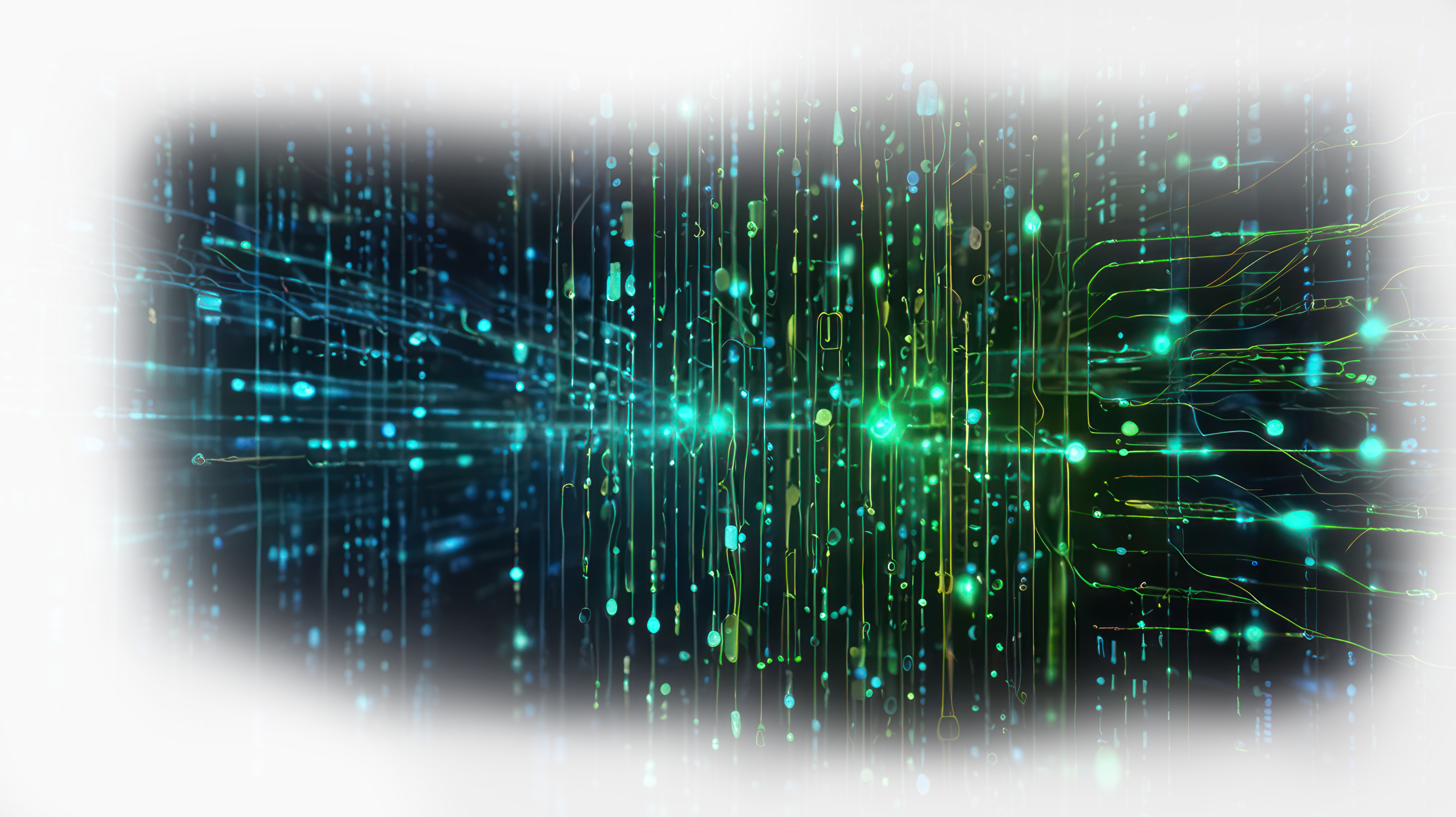
Target Audience
Designed for individuals looking to expand their expertise in machine learning, this course is perfect for data analysts, data scientists, and data engineers aiming to get hands-on exposure to the latest ML tools on Google Cloud such as Vertex AI AutoML, BQML, Dataflow, and more
What you'll learn
Build, train, and deploy ML models with Vertex AI AutoML and manage datasets in Vertex AI.Use Vertex Vizier for hyperparameter tuning and Feature Store for efficient feature management.Implement custom training with TensorFlow/Keras and optimize models using loss functions and metrics.Perform EDA, improve data quality, and create scalable datasets for training and evaluation.Leverage Vertex AI Pipelines to automate and scale the ML workflow.
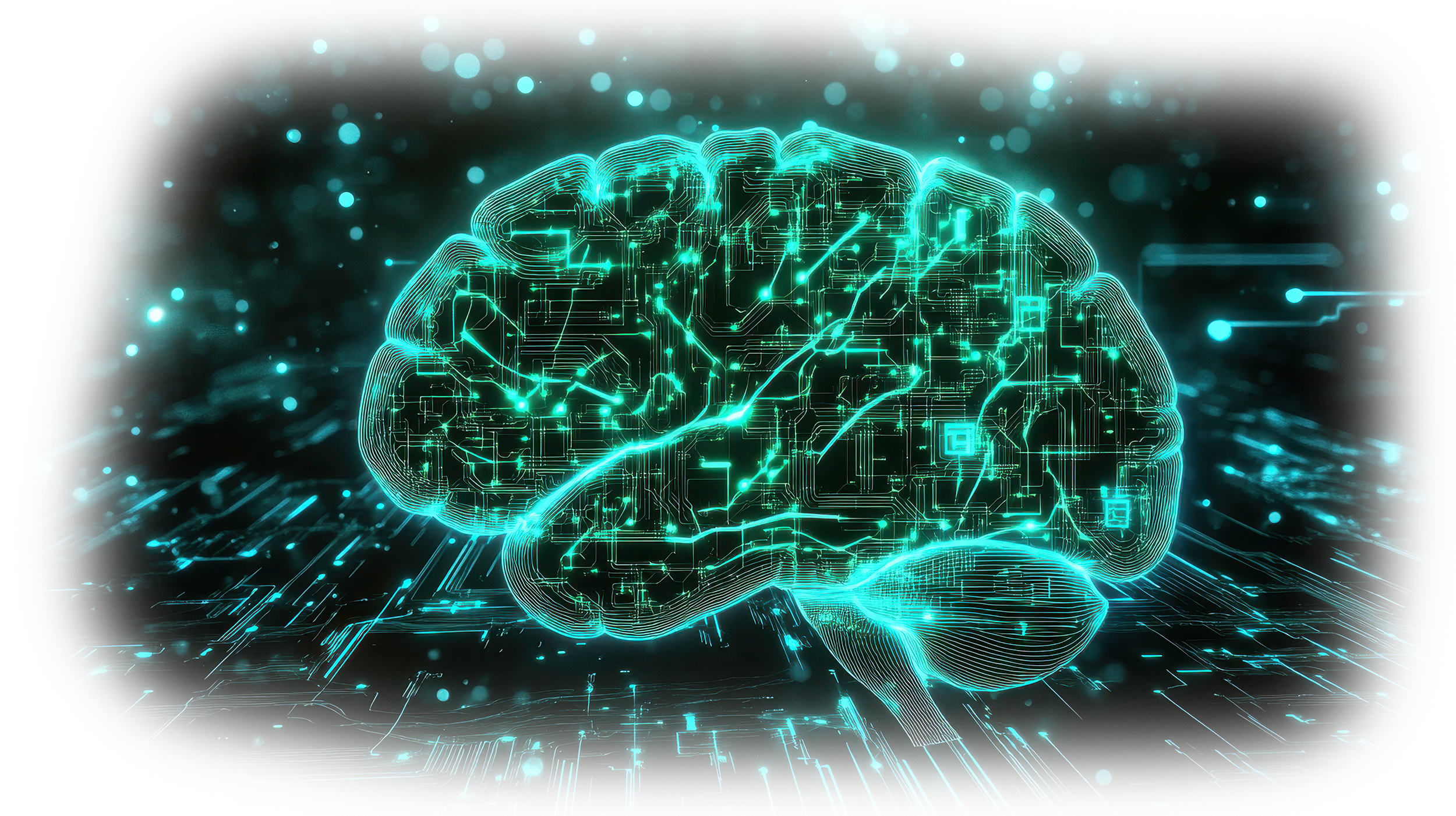
Prerequisites for Success
Prerequisites for Success
Participants should have basic machine learning knowledge and basic Python proficiency to get the most out of this course.
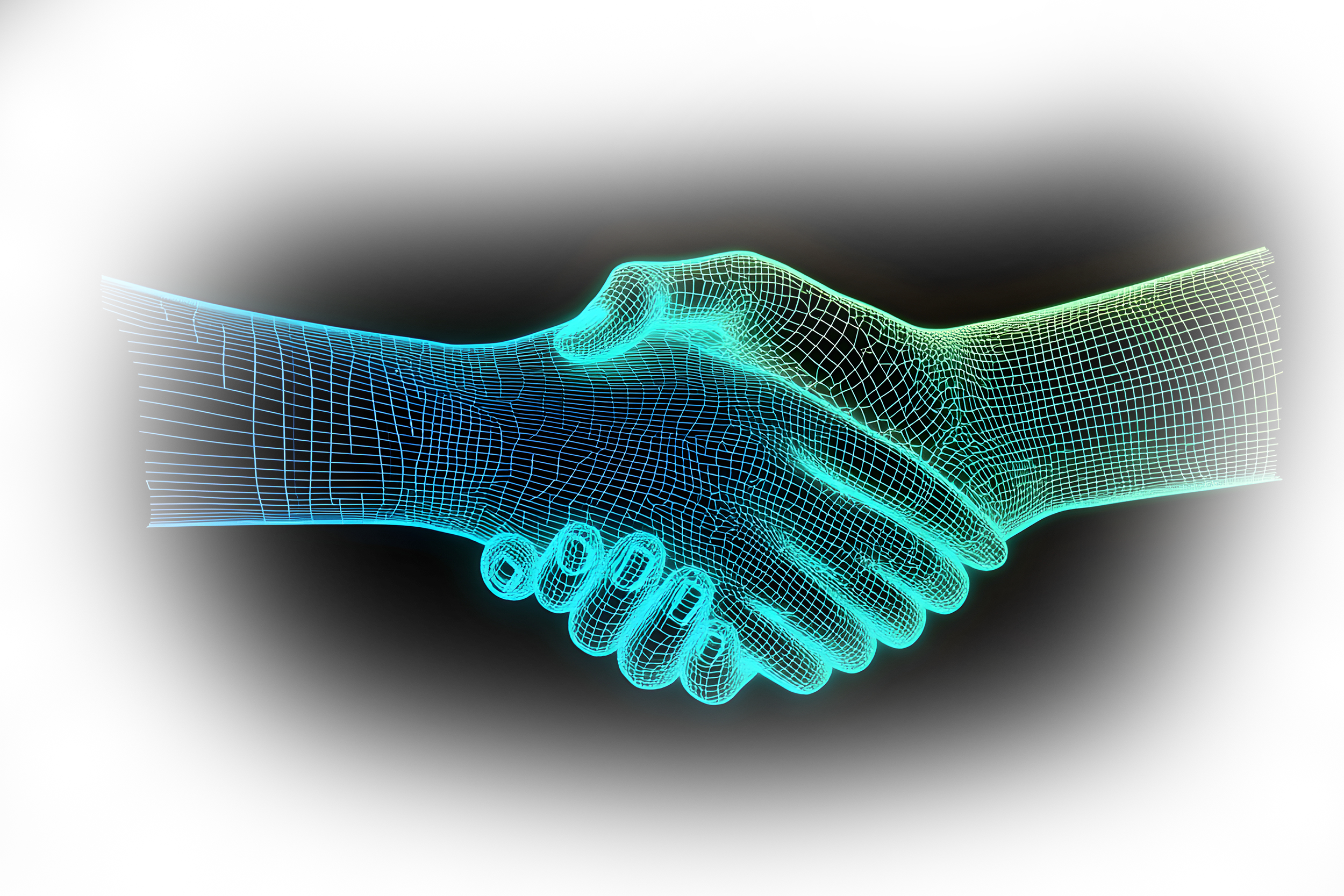
COURSE AGENDA
How Google Does Machine Learning
- Vertex AI Platform Overview: Understand how Vertex AI is used to quickly build, train, and deploy AutoML models without writing a single line of code.
- Best Practices for Implementing ML on Google Cloud: Learn the best practices for implementing machine learning on Google Cloud, ensuring efficiency and scalability.
organization. - Reimagining Use Cases through ML: Examine real-world use cases and explore how they can be reimagined through the lens of machine learning.
- Leveraging Google Cloud Tools for ML: Discover how to use the wide array of Google Cloud tools and environments for successful ML implementation.
Launching into Machine Learning
- Improving Data Quality: Learn how to improve data quality to ensure effective training of machine learning models.
- Exploratory Data Analysis (EDA): Perform exploratory data analysis (EDA) to better understand your dataset before building models.
- BigQuery ML Overview: Discover BigQuery ML and its benefits for building and training models directly in BigQuery using SQL.
- Supervised Learning Models: Learn how to build and train supervised learning models, essential for classification and regression tasks.
TensorFlow on Google Cloud
- Creating ML Models with TensorFlow and Keras: Learn how to create machine learning models using TensorFlow and Keras, two leading ML frameworks.
- Key TensorFlow Components: Understand the key components of TensorFlow and how to leverage them in model development.
- Using tf.data for Large Datasets: Learn how to use tf.data for efficiently manipulating and processing large datasets.
Feature Engineering
- Vertex AI Feature Store: Discover the Vertex AI Feature Store and how it enables efficient management of features for machine learning models.
- Key Aspects of a Good Feature: Compare the key aspects that make a feature valuable for machine learning.
- Feature Crosses: Learn how to create new features through feature crosses to improve model performance.
Machine Learning in the Enterprise
- Data Management and Governance Tools: Learn about the essential tools for data management and governance in machine learning workflows.
- Best Data Preprocessing Practices: Understand the best approach for data preprocessing, using tools like Dataflow and Dataprep for scalable, efficient preprocessing tasks.
- Choosing the Right Framework for ML: Learn the differences between AutoML, BigQuery ML, and custom training, and when to use each framework for specific tasks.
- Hyperparameter Tuning with Vertex Vizier: Explore how Vertex Vizier helps with hyperparameter tuning, improving model performance.